Timeseries data¶
In this example we calculate the data of a wind farm with 67 turbines in a time series containing 8000 uniform inflow states.
The required imports are:
In [1]:
%matplotlib inline
import matplotlib.pyplot as plt
import foxes
import foxes.variables as FV
import foxes.constants as FC
First, we create the states
. The data_source
can be any csv-type file (or pandas
readable equivalent), or a pandas.DataFrame
object. If it is a file path, then it will first be searched in the file system, and if not found, in the static data. If it is also not found there, an error showing the available static data file names is displayed.
In this example the static data file timeseries_8000.csv.gz
will be used, with content
Time,ws,wd,ti
2017-01-01 00:00:00,15.62,244.06,0.0504
2017-01-01 00:30:00,15.99,243.03,0.0514
2017-01-01 01:00:00,16.31,243.01,0.0522
2017-01-01 01:30:00,16.33,241.26,0.0523
...
Notice the column names, and how they appear in the Timeseries
constructor:
In [2]:
states = foxes.input.states.Timeseries(
data_source="timeseries_8000.csv.gz",
output_vars=[FV.WS, FV.WD, FV.TI, FV.RHO],
var2col={FV.WS: "ws", FV.WD: "wd", FV.TI: "ti"},
fixed_vars={FV.RHO: 1.225},
)
We can visualize the wind distribution via the StatesRosePlotOutput
. Here we display the ambient wind speed in a wind rose with 16 wind direction sectors and 5 wind speed bins:
In [3]:
o = foxes.output.StatesRosePlotOutput(states, point=[0., 0., 100.])
fig = o.get_figure(16, FV.AMB_WS, [0, 3.5, 6, 10, 15, 20], figsize=(6, 6))
plt.show()
Selecting 'DefaultEngine(n_procs=15, chunk_size_states=None, chunk_size_points=20000)'
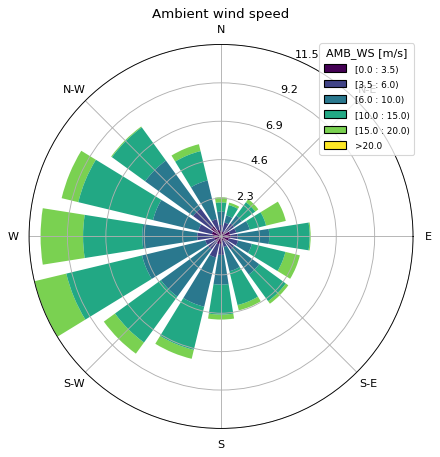
For the time series with uniform data, any choice of the point
argument will produce the same figure.
Next, we create the example wind farm with 67 turbines from static data. The file test_farm_67.csv
has the following structure:
index,label,x,y
0,T0,101872.70,1004753.57
1,T1,103659.97,1002993.29
2,T2,100780.09,1000779.97
3,T3,100290.42,1004330.88
...
For more options, check the API section foxes.input.farm_layout
.
We consider two turbine models in this example: the wind turbine type NREL5MW
and the turbine model kTI_02
, both from the default model book. The latter model adds the variable k
for each state and turbine, calculated as k = kTI * TI
, with constant kTI = 0.2
. The parameter k
will later be used by the wake model.
In [4]:
farm = foxes.WindFarm()
foxes.input.farm_layout.add_from_file(
farm, "test_farm_67.csv", turbine_models=["NREL5MW"], verbosity=0
)
Next, we create the algorithm
, with further model selections. In particular, two wake models are invoked, the model Bastankhah_quadratic
for wind speed deficits and the model CrespoHernandez_max
for turbulence intensity:
In [5]:
algo = foxes.algorithms.Downwind(
farm,
states,
rotor_model="centre",
wake_models=["Bastankhah2014_quadratic_ka02", "CrespoHernandez_max_ka04"],
verbosity=0,
)
Also notice the chunks
parameter, specifying that always 1000 states should be considered in vectorized form during calculations. The progress is automatically visualized when invoking the DaskRunner
:
In [6]:
farm_results = algo.calc_farm()
fr = farm_results.to_dataframe()
print("\n", fr[[FV.WD, FV.AMB_REWS, FV.REWS, FV.AMB_P, FV.P]])
WD AMB_REWS REWS AMB_P P
state turbine
2017-01-01 00:00:00 0 244.06 15.62 15.610500 5000.00 5000.000000
1 244.06 15.62 14.012903 5000.00 5000.000000
2 244.06 15.62 15.021033 5000.00 5000.000000
3 244.06 15.62 15.560020 5000.00 5000.000000
4 244.06 15.62 14.564008 5000.00 5000.000000
... ... ... ... ... ...
2017-06-16 15:30:00 62 299.19 11.70 8.145984 4868.75 1880.222715
63 299.19 11.70 11.490711 4868.75 4777.186188
64 299.19 11.70 11.700000 4868.75 4868.750000
65 299.19 11.70 11.642754 4868.75 4843.705093
66 299.19 11.70 8.055085 4868.75 1812.276034
[536000 rows x 5 columns]
Let’s evaluate the results:
In [7]:
# add capacity and efficiency to farm_results:
o = foxes.output.FarmResultsEval(farm_results)
o.add_capacity(algo)
o.add_capacity(algo, ambient=True)
o.add_efficiency()
# print results by turbine:
turbine_results = o.reduce_states(
{
FV.AMB_P: "mean",
FV.P: "mean",
FV.AMB_CAP: "mean",
FV.CAP: "mean",
FV.EFF: "mean",
}
)
turbine_results[FV.AMB_YLD] = o.calc_turbine_yield(algo=algo, annual=True, ambient=True)
turbine_results[FV.YLD] = o.calc_turbine_yield(algo=algo, annual=True)
print("\nResults by turbine:\n")
print(turbine_results)
# print power results:
P0 = o.calc_mean_farm_power(ambient=True)
P = o.calc_mean_farm_power()
print(f"\nFarm power : {P/1000:.1f} MW")
print(f"Farm ambient power: {P0/1000:.1f} MW")
print(f"Farm efficiency : {o.calc_farm_efficiency():.2f}")
print(f"Annual farm yield : {turbine_results[FV.YLD].sum():.2f} GWh")
Capacity added to farm results
Ambient capacity added to farm results
Efficiency added to farm results
Results by turbine:
AMB_P P AMB_CAP CAP EFF AMB_YLD \
turbine
0 3067.723397 2638.907209 0.613545 0.527781 0.774911 26.873257
1 3067.723397 2299.506817 0.613545 0.459901 0.631419 26.873257
2 3067.723397 2564.420645 0.613545 0.512884 0.730530 26.873257
3 3067.723397 2609.874904 0.613545 0.521975 0.760051 26.873257
4 3067.723397 2392.958531 0.613545 0.478592 0.664594 26.873257
... ... ... ... ... ... ...
62 3067.723397 2457.791530 0.613545 0.491558 0.687892 26.873257
63 3067.723397 2435.429893 0.613545 0.487086 0.673638 26.873257
64 3067.723397 2756.583392 0.613545 0.551317 0.825891 26.873257
65 3067.723397 2383.050318 0.613545 0.476610 0.656621 26.873257
66 3067.723397 2408.628197 0.613545 0.481726 0.672845 26.873257
YLD
turbine
0 23.116827
1 20.143680
2 22.464325
3 22.862504
4 20.962317
... ...
62 21.530254
63 21.334366
64 24.147671
65 20.875521
66 21.099583
[67 rows x 7 columns]
Farm power : 166.3 MW
Farm ambient power: 205.5 MW
Farm efficiency : 0.81
Annual farm yield : 1456.42 GWh
We can visualize the mean rotor equivalent wind speed as seen by each turbine and also the mean efficiency with respect to the time series data as colored layout plots:
In [8]:
fig, axs = plt.subplots(1,2,figsize=(14,5))
o = foxes.output.FarmLayoutOutput(farm, farm_results)
o.get_figure(fig=fig, ax=axs[0], color_by="mean_REWS", title="Mean REWS [m/s]", s=150, annotate=0)
o.get_figure(fig=fig, ax=axs[1], color_by="mean_EFF", title="Mean efficiency [%]", s=150, annotate=0)
plt.show()
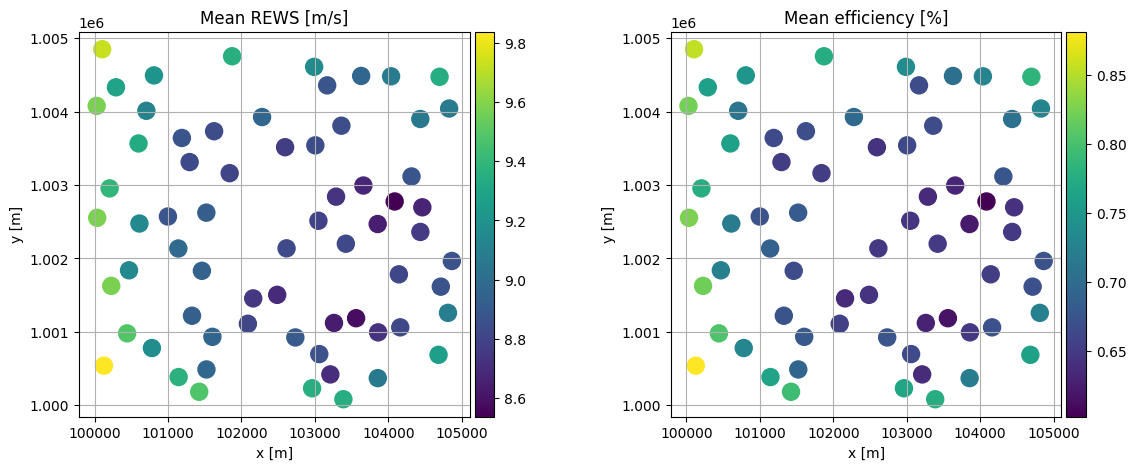